Quo vadis, quantum machine learning?
Quantum-based machine learning is showing progress in efficiency and generalization, but clear advantages over classical methods for unstructured data are still lacking. Focus on challenges and opportunities.
Quantum machine learning holds significant promise for enhancing various aspects of machine learning, including sample complexity, computational complexity, and generalization. The field has made substantial strides in recent years. However, a key objective—developing quantum algorithms that clearly outperform classical methods for practically relevant unstructured data—remains elusive. In this talk, we will explore this challenge from multiple perspectives, avoiding any hype or over-exaggereation. We will examine cases where separations can be identified, such as in abstract instances of generator [1] and density modeling [2], in training classical networks using quantum algorithms [3], for short quantum circuits [4], and for quantum analogs of diffusion probabilistic models [5]. At the same time, we will address challenges arising from dequantization in both noise-free [6] and non-unital noisy settings [7]. These insights will also encourage thinking beyond traditional approaches. We will reconsider the concept of generalization [8] and explore examples of explainable quantum machine learning [9] and single-shot quantum machine learning [10]. Ultimately, we will use these insights to reflect on the potential and limitations of applying quantum computers to machine learning problems involving unstructured noisy data.
[1] Quantum 5, 417 (2021).
[2] Phys. Rev. A 107, 042416 (2023).
[3] Nature Comm. 15, 434 (2024).
[4] arXiv:2411.15548 (2024).
[5] arXiv:2502.14252 (2025).
[6] Quantum 9, 1640 (2025).
[7] arXiv:2403.13927 (2024).
[8] Nature Comm. 15, 2277 (2024).
[9] arXiv:2412.14753 (2024).
[10] arXiv:2404.03585 (2024).
Presentation language: EN
Speakers (1)
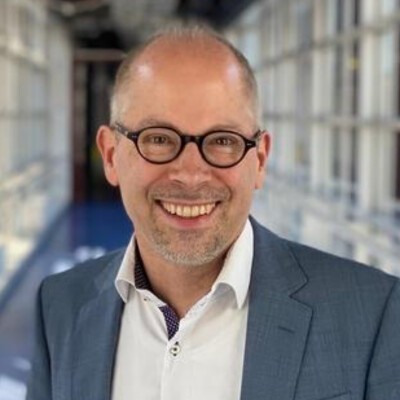